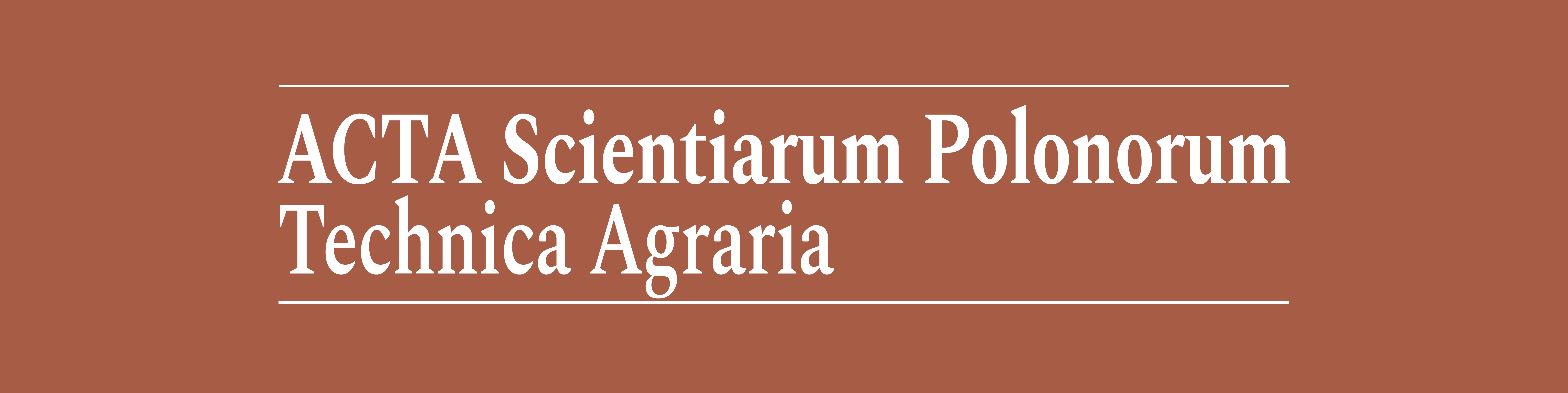
W pracy przedstawiono bazujący na metodzie Prony'ego algorytm identyfikacji modułu relaksacji liniowych materiałów lepkosprężystych opisanych modelem Maxwella. Udowodniono, że jeśli rzeczywisty moduł relaksacji dany jest czteroelementowym modelem Maxwella, a jego pomiary nie są obciążone zakłóceniami, to algorytm ten zapewnia identyfikowalność rzeczywistych parametrów modułu relaksacji. Pokazano, że wszystkie zadania obliczeniowe algorytmu są dobrze postawione w sensie Hadamarda i podano konieczne i dostateczne warunki stosowalności algorytmu do zadania identyfikacji czteroelementowych modeli Maxwella. Efektywność algorytmu zilustrowano wyznaczając funkcję relaksacji naprężeń próbki korzenia buraka cukrowego w warunkach stanu jednoosiowego odkształcenia oraz dla danych Lanczosa.
Możesz również Rozpocznij zaawansowane wyszukiwanie podobieństw dla tego artykułu.