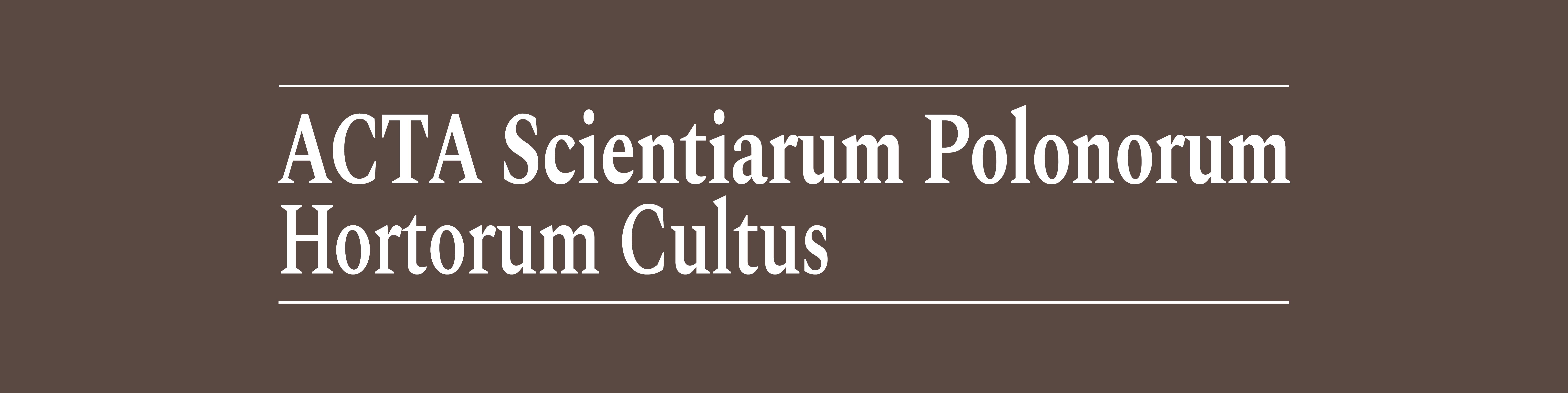
An image analysis method based on RGB features for rapid estimation of the chlorophyll content of leaves of micropropagated strawberry plants (2 cultivars) was presented in the study. An algorithm describing the relationship between the absolute values of chlorophyll content and the colour components of a leaf image captured with a conventional scanner was developed and tested. The accuracy of the proposed method was compared with that of an optical greenness meters designed for assessing leaf chlorophyll content. The chlorophyll content in the strawberry leaves was correlated with the results of measurements recorded by the two optical meters (SPAD-502, CCM-200) and with RGB values of scans of these leaves. The highest values of correlation coefficients were obtained between the chemical analysis results and mean values of the red colour (R) of the scans. However, varietal differences were evident here, which indicates the need for individual calibrations. In the case of the green colour (G), the accuracy was slightly lower; however, no varietal differences were found, thus one calibration can be used for both cultivars. Three formulas: (R-G)/(R+G), (R-G)/(R+G+B), and R/(R+G+B) were selected and their relationship with the changes in chlorophyll content was tested. These variables did not explain the changes in chlorophyll content better than the variable R. The study confirmed the possibility of using the image capture method for the detection of chlorophyll status in strawberry plantlets cultured in vitro.
You may also start an advanced similarity search for this article.